Detailansicht
Accessing the Hidden Space with Explainable Artificial Intelligence | Dr. Sebastian Lapuschkin (Fraunhofer Heinrich Hertz Institute, HHI Berlin)
Abstract:
The emerging field of explainable Artificial Intelligence (XAI) aims to bring transparency to today’s powerful but opaque deep learning models. However, the vast majority of current approaches to XAI only provide partial insights and leave interpreting the model’s reasoning to the user. This talk will provide an overview on how established techniques from XAI can be used to successfully understand, debug and improve machine learning models and pipelines. The session will provide an outlook on how recent developments towards concept-based XAI (with a focus on the recent Concept Relevance Propagation (CRP) approach) will lead to more human interpretable explanations and thus enable novel analyses to gain insights into the reasoning of AI.
---
Short Bio:
Sebastian Lapuschkin received the Ph.D. degree with distinction from the Berlin Institute of Technology in 2018 for his pioneering contributions to the field of Explainable Artificial Intelligence (XAI) and interpretable machine learning. From 2007 to 2013 he studied computer science (B. Sc. and M. Sc.) at the Berlin Institute of Technology, with a focus on software engineering and machine learning. Currently, he is the Head of the Explainable Artificial Intelligence at Fraunhofer Heinrich Hertz Institute (HHI) in Berlin. He is the recipient of multiple awards, including the Hugo-Geiger-Prize for outstanding doctoral achievement and the 2020 Pattern Recognition Best Paper Award. His work is focused on pushing the boundaries of XAI, e.g, for achieving human-understandable explanations, or towards the utilization of interpretable feedback for the improvement of machine learning systems and data. Further research interests include efficient machine learning and data analysis, data and algorithm visualization.
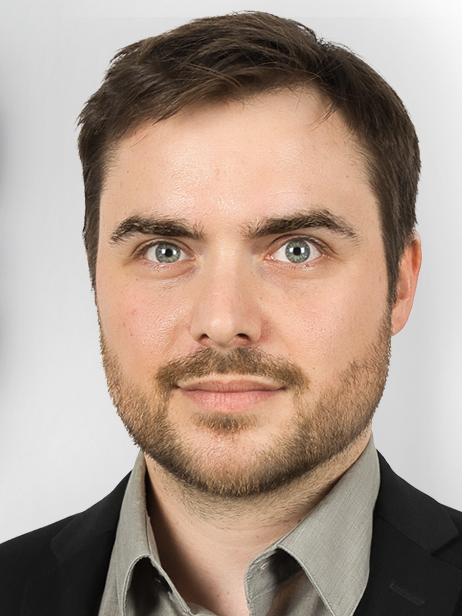